Allegations of cheating online have been making the headlines again, but there are emerging high-tech solutions that can make poker safe for everyone. A5 Labs – who describes itself as “a team of tech entrepreneurs, AI scientists, online game operators, and die-hard fans, committed to shaping the future of online competitive games” – has put together an extensive white paper “to build the technology foundation and solutions for the next generation of online competitions”. Poker.Org is proud to bring you this paper, in full, that sets out the issues that online poker faces, and details the solutions that will see safe, fair and fun games dominate in the future.
Author: Thanh Tran comes with a background as a professor, entrepreneur and executive. He led a worldwide AI research group during his tenure as professor at KIT/Germany. After relocating to the Bay Area and a brief stint at Stanford, he went on to help StartX companies with their tech and funding raising as well as build his own companies in the area of Semantic Search, Smart Virtual Assistant and Data-/AI-driven Health Care. As an engineering executive, he helped Upwork successfully go public and, shortly after, decided to pursue his passion in poker. Currently, he is co-CEO of A5 Labs and a broader group of companies, committed to make poker and online competitive games more high-tech, safe, fair and fun.
The white paper was reviewed by A5 Labs staff and created with editorial help from John Andress (Head of Security Solutions), Vitalii Rizol (Head of Design) and Yongtao Ma (Head of Data).
Advanced Technologies for Secure, Fair and Fun Online Poker
Recent high-profile cheating scandals have shown that online poker has come a long way. Operators are thriving, successful players are earning tens of millions of dollars per year, and there is a vibrant community of good actors and powerful opinion leaders helping to steer online poker in the right direction.
However, they also revealed that online poker seems low tech, suffering from security and fairness issues, especially collusion and the non-legitimate use of bots and Real-Time Assistance (RTA). Apart from a few sites that are leading the way in their commitment and investment towards game integrity, it seems online poker is less fun, especially for recreational players, due to the dominance of predatory actors.
Security and Fairness in Online Poker
Imagine you’re playing a game of poker where one player sitting on your left is ghosting, the two on your right are colluding, and the remaining players are bots or playing essentially just like bots by using RTA. On top of that you’re wondering if the operator is employing an unbiased RNG and applying all the required security standards such as player hand encryption. There is a lot of terminology to unpack here but what it means is you’re playing a rigged game with a low chance of winning.
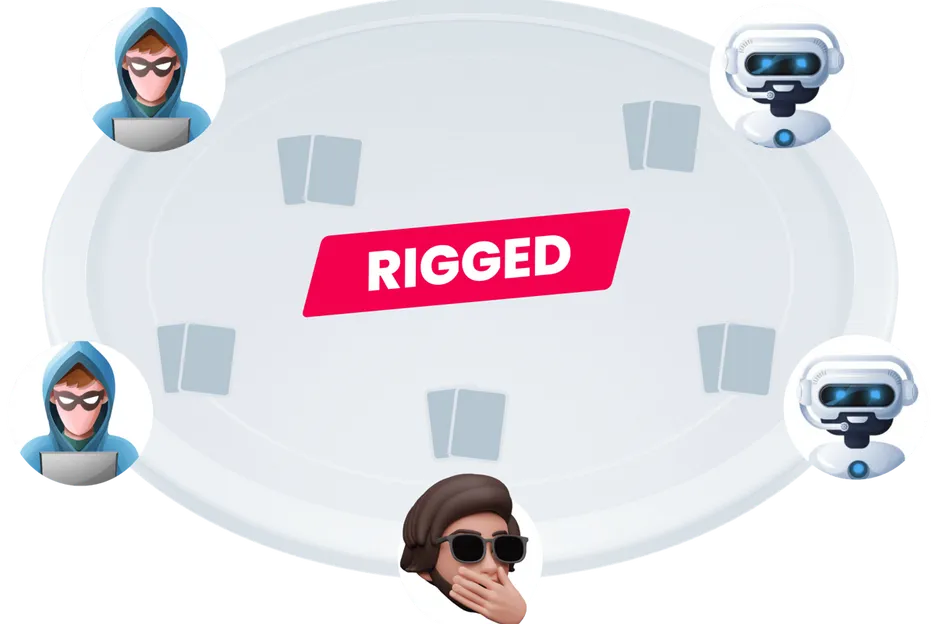
- RNG
Acronym for the random number generator that is the backbone of fair poker. There are different sources of randomness that can be used as seed (such as amplitude of atmospheric pressure) and independent auditors (like BMM Testlabs and GLI) provide certificates to operators when they can provably demonstrate the card sequences used in their games are random. - Data encryption
Using standards such as SSL, operators can make sure personal, financial and gameplay data from customers is being encoded as gibberish, such that anyone who may intercept it would need a key to make sense of it. - Collusion
Broadly, a type of gameplay involving multiple users taking illegitimate actions for their own benefit. A very common example of colluding is card sharing. Knowing the cards of another player helps to better calculate odds, and to use them as blockers for inferring other players' actions. - Ghosting
When a player receives real-time advice from another player on how to play their hands. - RTA
When a player receives real-time advice from software. Typically, this software is built on a database of solutions generated by a Game Theory Optimal (GTO) solver. Given the current hand, the software retrieves and provides assistance based on the best matching GTO solution. - Poker Bot
Software capable of mimicking a player that, using a data scraper plus GTO and/or Machine Learning based decision engines, takes actions without human supervision.
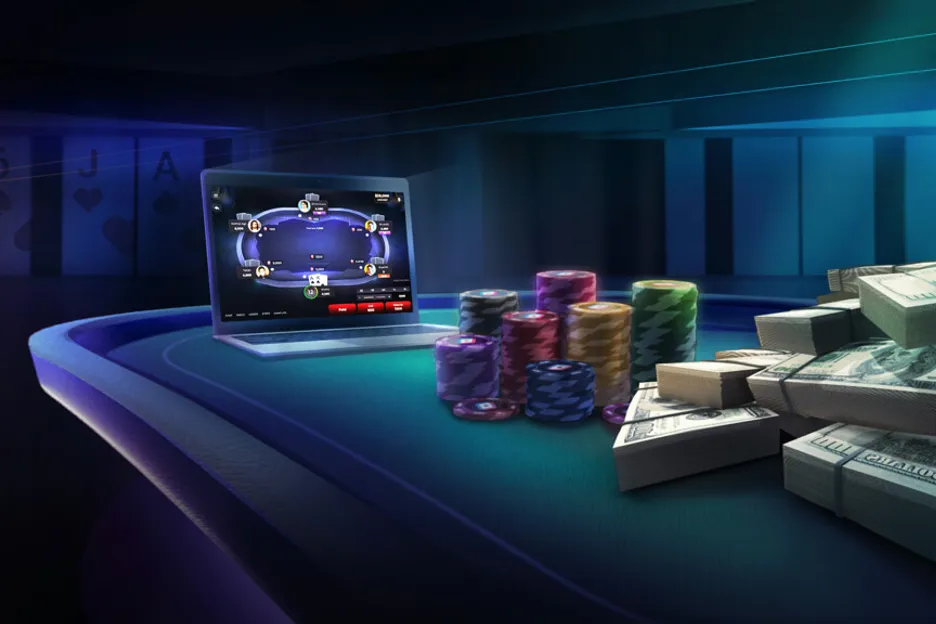
Poker Is and Should Be Fun
Beyond security and fairness, what really drives players to brick-and-mortar casinos is entertainment. There is a sizable community of poker players who love the technical aspect of the game. They are motivated by the competition, by the opportunity to face and show off their ability against other skilled players. But, by and large, the industry thrives and is largely dependent on the recreational audience that wants to see action, excitement and entertaining gameplay. However, the majority of poker operators today are driven by short-term revenue, mainly attracting the professional (and often predatory) players that have a bigger impact on engagement and their bottom line. Recreational users find tough games and face strong competition they cannot beat.
We believe online poker is only viable in the long term when operators are able to provide an experience where users feel secure and safe, know the game is fair, and where there is plenty of action for the recreational audience who have a chance of winning and feeling entertained.
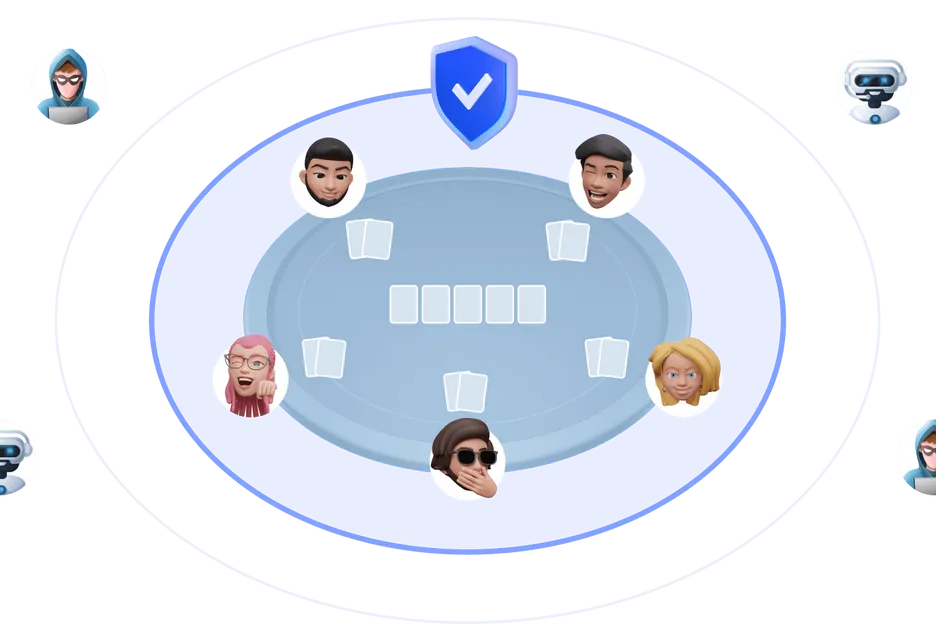
Advanced Tech for the Growing Poker Industry
Being part of a team of leading AI/GTO experts, elite poker players and successful tech business owners with a passion for poker, we are optimistic about the future for the following reasons:
- We believe recent widespread game integrity concerns should be viewed as a signal of success. It is a strong indication poker has reached the penetration and maturity we have seen in other industries. When an industry is gaining significant traction, it means there is Big Money, and money attracts advanced cheaters and foul play. But money also drives innovations and produces successful solutions to combat all growth issues.
- Understanding that secure, fair and fun games are essential for long-term sustainability, there are operators in the industry that have made significant investment and achieved tremendous progress in the past few years. We have seen extensive crackdown of bot rings, and the signatures of cheaters and predatory behavior being constantly updated to adapt to new changes in foul play. Through the severe punishment and early identification, we see cheaters cannot turn a profit at scale. We see operators applying technologies to infer the skill levels of players using fewer than 200 hands, and successfully helping their recreational users to find games that are a better match for their level, games where they have a fairer chance of winning. We see operators devising smart incentive schemes to ensure their professional players continue to make a strong profit as long as they contribute to engagement, fun, action, and the overall sustainability of the ecosystem.
- Technology is the answer to hard problems and some major operators are very advanced in their technical roadmap towards ensuring high game integrity and sustainable online poker. Game integrity issues and security-related topics at large, however, are more taboo because it seems there is little for operators to gain in addressing these in public. On the contrary, we believe the next level of advancements can only be achieved when operators come clean and step out of the dark. Individual operators will be more successful when they share information and work together towards commonly accepted security standards, enabling third-party audits to reveal and certify their success, and overall, being more transparent to users to instill confidence and trust in their capabilities.
In more detail, we will now discuss the adoption of modern technologies and outline a long-term term roadmap we believe will help not only individual operators but the industry at large to ensure secure, fair, and fun experiences for online poker users.
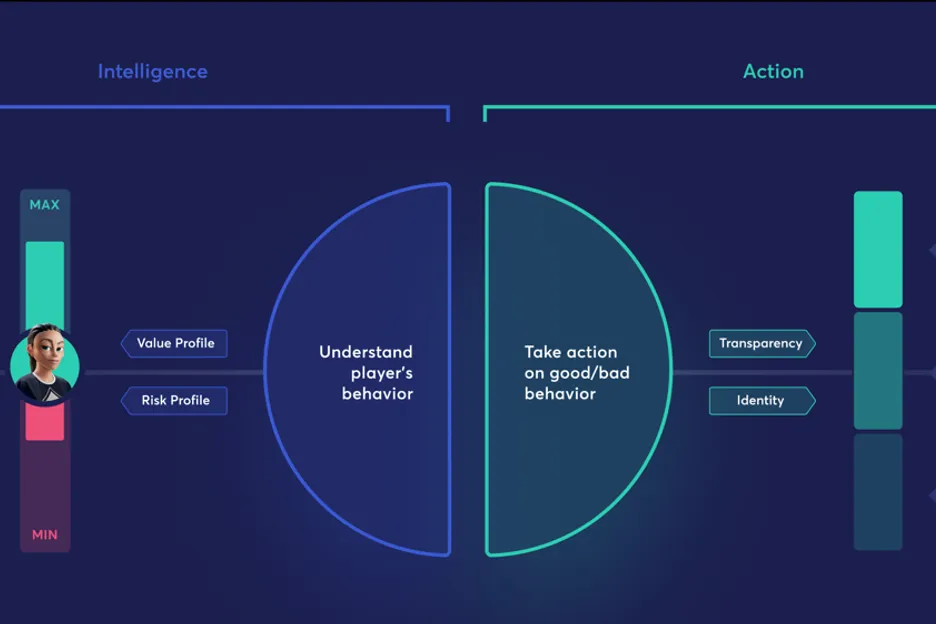
Core Pillars of Secure, Fair and Fun Online Poker Tech
The tech foundation for sustainable poker is to know the players, to be able to quantify positive and negative behavior, and to be transparent about the underlying mechanics. The impact of this is especially amplified when information is made available publicly such that good actors benefit and bad actors get penalized across sites.
- Value Profile
Operators care about how much players impact their bottom line. But this traditional approach focusing on revenue contribution is short-sighted, resulting in short-term gains that come at the cost of long-term viability. The challenge is to quantify and factor in positive behaviours that add action, excitement and entertainment value. We believe an essential, but often under-appreciated, trait of high integrity and high value poker players is their willingness to compete in “tough” games. These players contribute to engagement at a much lower cost to the health of the ecosystem (as opposed to “bumhunters”) and, hence, should receive credit points to their value profile. - Risk Profile
This is the most underdeveloped capability because, largely, operators are geared towards maximizing revenue no matter the risk. Fortunately, regulated businesses can only acquire and maintain gaming licenses when they adhere to compliance standards. Licensed operators successfully managing these compliance risks have minimum solutions to Know Your Customers (KYC), track deposits and withdrawals to comply with Anti Money Laundering (AML) regulations, and identify excessive sessions and spending to enforce Responsible Gaming. While risk management often ends here, we believe sustainable poker operators must extend their scope beyond protecting themselves to protecting their players. The challenge is to quantify bad behaviors that have a negative impact on the experience of other players including collusion, rat-holing, hit and running, buttoning, and the use of RTA and bots. - Identity
Knowing the players is easy and this is something individual operators do well when adopting widely available KYC tools and services. But KYC adds friction to the conversion funnel so operators shy away from excessive adoption unless it is required for the sake of compliance. What is more common is the use of player signatures that are derived from contextual information and gameplay. Beyond the challenge of computing a reliable signature to identify players, the bigger problem/opportunity is industry-wide adoption. If the entire industry had access to a public shared database of player identities, more effective deterrence can be established against bad actors and greater benefits can be provided to good actors. High-value users can enjoy better bonuses and greater rewards while players with a higher risk profile are limited from accessing games and events, both online and offline. We believe the key to this is a concept of anonymous identity – how do we share the identification of players without sacrificing anonymity, without revealing their identity publicly? - Transparency
Transparency here is two-fold:
(1) First is for operators to embrace technical means that allow them to share information with one another to amplify the impact of their efforts.
(2) Second is to educate the public, reveal solutions they have in place to users, and work towards commonly accepted standards that enable third-party audits to certify not only their RNG but all security related procedures and solutions. At the core of this, what will drive transparency is consensus on the evidence used to establish value and risk behaviors. Take credit scoring as an example: a few major ones have emerged as the de facto standard, and whether it is FICO or any other, it is transparent and clear as to what factors influence the credit score.
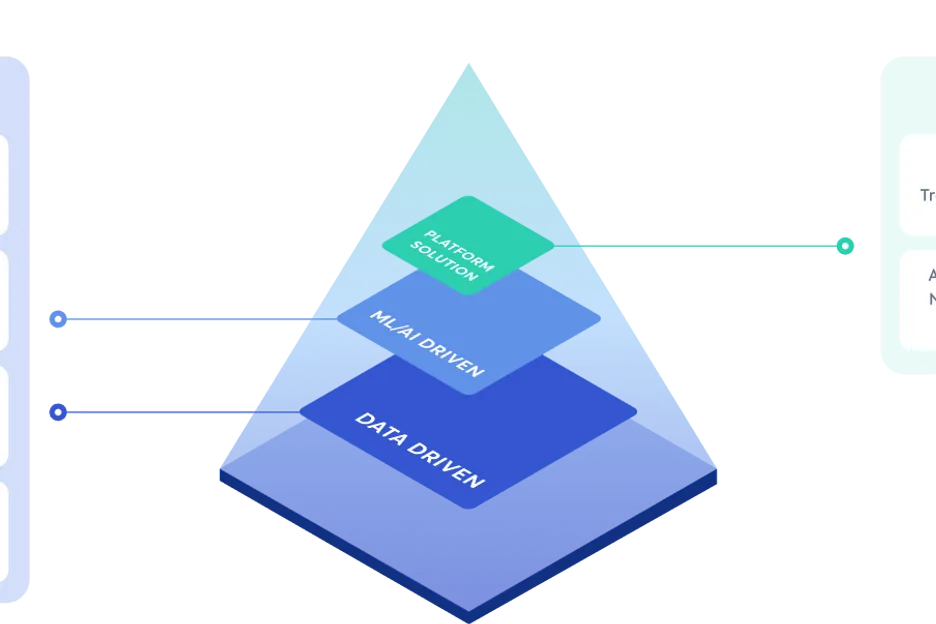
A Technical Roadmap for Advanced Poker Tech
So where are we now in building these core pillars and creating the technology solutions we need for poker to thrive? We will highlight some of the advancements we have seen in action as well as discuss future directions towards a longer-term roadmap.
The Foundation: Data-driven Solutions
The foundation of the poker tech stack is the data layer. This comprises: (1) a batch data infrastructure that allows data to be stored, processed in batches and managed at scale, (2) stream data processing (3) analytics capabilities to drive insights, generate recommendations, and make real-time decisions. On top of this sits trackers that follow users throughout all their interactions with the system, collecting contextual data, their use of softwares, their actions and gameplay.
Using data collected this way, operators employing what we refer to as “basic” solutions are able to gain a rudimentary understanding of their player’s identity, risk and value profile:
- Contextual identity: device, network, location, personal information (email, phone number, address, bank and credit card information).
- Value profile based on common metrics: cost of acquisition, contribution to engagement and revenue, estimated customer lifetime value based on revenue contribution, rudimentary bucketing of players into value groups (Action Player, Fish, Whales, Pros) based on game stats (Voluntarily Put $ in Pot, Preflop Raise, Postflop Aggression Frequency, Big blinds won/100 hands).
- Risk profile based on the use of illegitimate software: VPN, screen share, data scraper, poker HUD, RTA, bot.
- Risk profile based on player’s behavior vs. known “risk patterns” manually established by domain experts: login and session patterns, deposit and withdrawal patterns, browsing and click patterns.
All major poker operators have some sort of solutions for data tracking. Larger operators have an edge in the metrics and patterns they are able to establish and keep up-to-date over time based on the amount of data they hold and the investment they can make in domain expertise. With these solutions, they are able to combat the lion’s share of foul play but there is still a long way towards gaining full user trust due to these drawbacks:
- Invasive
Tools used for tracking the use of prohibited software, monitoring processes, and in extreme cases recording the customer’s screen. Players are advised to check the T&Cs of poker operators and make sure their adoption of these tools does not include spywares and their practices do not undermine users’ privacy. - Too much data, too few insights
The amount of available data is extremely large and noisy, especially behavior and gameplay-related information. A common challenge in Big Data analysis is Confirmation Bias. The tendency to search and interpret information in a way that supports one’s belief is exacerbated by the reliance on domain experts and manually encoded patterns. Given a large dataset, it is easy to find behavior poker experts deem problematic, but it is very difficult to quantify the risk and make reliable decisions. - Easy to circumvent
Another problem with manually encoded knowledge, or patterns that in any sort of form can be made explicit, is circumvention. Simply based on the discussion above, sophisticated poker players can already tell what kinds of patterns operators are looking for and how simple modification to gameplay and behavior can help bypass the system.
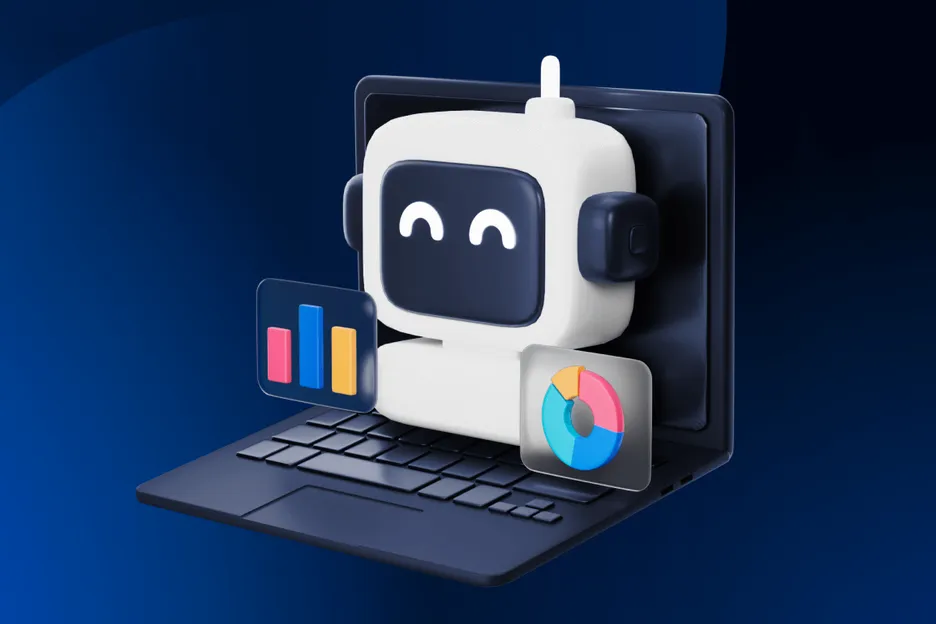
The Frontier: AI-/ML-/Intelligence-driven Solutions
We think “basic” solutions in the above category are already having a large impact on keeping foul play in check. Complementing this partial solution are advances in Game Theory, Artificial Intelligence (AI), and Machine Learning (ML) that help turn data into actionable insights and enable automated decisions players cannot circumvent at much greater cost.
GTO Solvers
GTO solvers are built on the Game Theory foundation of poker. In theory, it is known the optimal poker strategy can be found in the Nash equilibrium where each player knows the optimal/equilibrium strategies of the other players, and no player can gain by changing their strategy. In simple terms, it means when playing a GTO strategy, a player becomes unexploitable and will make a profit when other players deviate from that strategy, i.e. when they make GTO mistakes.
Using solvers, we can establish a GTO baseline and use it to quantify GTO deviations/mistakes in every player’s strategy. We can answer questions such as how close a player is playing from the optimal strategy, and where there were deviations, did it result in an unexpected gain for other players at the same table. This is very valuable in identifying RTA and bot usages as well as collusion among players. In practice, this is not straightforward for multiple reasons.
- It is computationally expensive to obtain the GTO baseline for every variation of the game (different game types, blind structures, etc.).
- Cheaters use simplifications and modifications to their setup to obtain near-GTO solutions that exhibit a great deal of mistakes, yet are profitable. Successful applications of this technology have shown that foul play is not simply based on the amount of mistakes but rather the severity/novelty/entropy of these mistakes. Even the best pros make many mistakes, and a lot of recreational players make relatively few mistakes in “common spots” such as preflop decisions. What uniquely identifies foul play is the ability to consistently make good decisions in infrequent spots that have severe consequences on profitability.
Machine Learning
Another complementary direction is to model game play using Machine Learning. GTO-based solutions still require a great deal of domain expertise and manual involvement of experts to be fully operational. ML-based solutions, on the other hand, have the potential to be plug-and-play, enabling automated decisions with little manual intervention.
Significant progress, especially in Deep Learning (DL), helped revolutionize the modeling and automated recognition of images, speech/utterances and languages. The idea is to automatically learn the underlying “latent” representation, aka features, to establish a task-independent/universal model that can be fine-tuned to specific tasks and goals.
With a language model, for example, we can predict the most sensible sentence completion given a few words, or the most probable answer given a question. Applied to poker, we can build a DL-based model using GTO gameplay data to predict the next GTO action given a sequence of actions and board texture. Using actual gameplay data and observed winnings, we can predict the most profitable action.
Given the participants, aka the competition, in a game, we can predict and quantify how well a given player will perform in that game in terms of BB won/100 hands. In general, the successful adoption of DL helps to establish powerful signatures of gameplay that can be used to enable a variety of inferences: whether a player belongs to a bot group, how similar their action is to known collusion behavior, or if their behavior is consistent across different sessions.
These “Frontier” solutions, aka the State-of-the-Art, are superior to their predecessors because they are non-invasive, produce reliable actionable insights and, especially due to their focus on gameplay, cannot be circumvented at a significant cost. Bad actors find it difficult to change their behavior without a negative impact on their profit. Without being particularly comprehensive, we’d like to highlight these successful applications of GTO and ML technologies:
- Value profile based on predicted lifetime value
Learn and predict future revenue contribution based on data capturing recency and amount of previous deposits, win and losses, and other in-game purchases. - Value profile based on contribution to ecosystem
Learn and predict positive impact on engagement, lifetime of other players as well as health and long-term sustainability of the ecosystem based on quantifying fun, action, and entertaining gameplay. For example, this can be achieved by predicting the duration of the game given a player joining or leaving the table. - Risk profile based on GTO mistakes
Establish indicators of RTA and bots using deviations and mistakes vs GTO baseline; infer other foul play such as collusion based on deviations from baseline resulting in unexpected gains for other players that often show up at the same table. - Risk profile based on machine-learned gameplay signature
Using latent representation derived from large-scale gameplay data to compute similarity, infer inconsistent gameplay, and detect affiliation with known bot groups or closeness to any sort of risk behavior. - Fair game adjustments based on inferred player skill level
Based on gameplay and winnings, infer skill level relative to opponents and provide suggestions for players to join tables that more closely match their level.
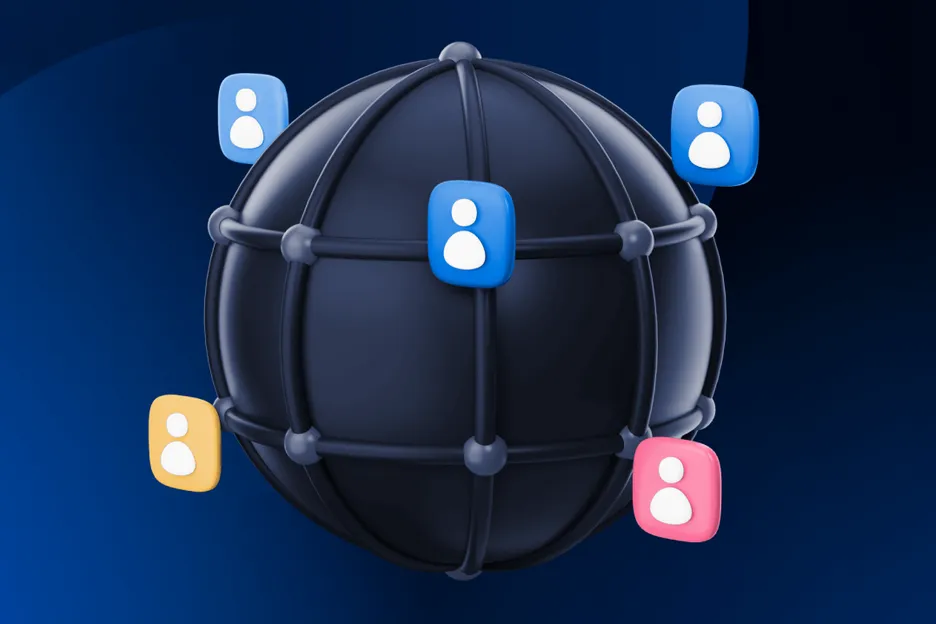
The Future: Holistic Platform Solution
We have covered solutions at the frontier of poker tech and it is based on their successful applications in practice that give us high confidence and optimism about the future of online poker. What remains to further eliminate the remaining micro-percents of bad actors and change the public perception is the work outside of the scope of what individual operators can do.
Furthermore, we believe to achieve the next stage of advancement, we have to rethink the platform and embrace more radical solutions. This is not an effort to preach Web3 because it is largely impractical for many industries to move away from the centralized power of Big Tech and there will be strong resistance from the few but large major players. However, we believe and argue that igaming, and especially the online poker industry, is a special case where the adoption of certain Web3 technologies is easier and will have large practical benefits to urgent problems.
As opposed to Big Tech, major online poker operators do not rely on monetizing and owning the user data, and they benefit more from sharing the data and being more transparent to gain user trust and confidence. As opposed to some industries where the battle might be already lost, online poker users are in a position of power. We see a future where poker operators will run out of business when they fail to compel users as to why their platform is secure, safe and fun. We will now discuss technologies and directions that will enable them to do so:
- Anonymous Identity based on the use of NFTs
With the use of Non-Fungible Tokens, aka NFTs, that can act as avatars for users in the online gaming world, users enjoy all the benefits of being identifiable while not sacrificing their anonymity. Rather than having to log into the system and providing all the personal information to verify their identity, a NFT avatar can act as a key to serve the same purpose. This will largely benefit players but also operators will see merits: good actors can build industry-wide reputation around their anonymous identity that – just like a healthy credit score – enable them to enjoy benefits similar to the current operator-specific loyalty points. Knowing their reputation, operators will be able to solve their “cold start” problem and customize their offerings to gain the most value from new customers. Users without an established reputation have to go through hoops such as KYC to fully gain access, and bad actors may find their real KYC identity being shared via blacklists that are enforced across operators/industry-wide. - Strong benefits tied to industry-wide NFT-based reputation
The adoption of NFTs as a reputation scheme will be greatly accelerated when there are strong incentives for users. Already today, VIP users enjoy outsized rewards when they are identified as big spenders with large revenue contributions. This is largely the case for casino games, but the concept of high-value users is poorly understood and incorrectly applied by online poker operators. Players who enjoy high rakeback are often limited to those putting in a huge amount of time irrespective of their “good” behavior and potential long lifetime. Given the advancements in modeling players’ value and positive long-term impact to the ecosystem, we see an opportunity for operators to revamp their platform and incentive scheme, and to also reward good and sustainable behavior that helps ensure their operations remain healthy in the long run. - Transparency based on open ledger, aka Blockchain
The concept of an open, distributed ledger that can record transactions in a verifiable and permanent way is very appealing in the pursuit of greater transparency. What makes an RNG trustworthy? What makes an operator’s risk-scoring decision more acceptable to the user? How can this information be shared and made publicly available (without compromising the real identity of players)? We have discussed various technology solutions that can be used to establish quantifiable indicators such as GTO mistake scores and similarity to known foul play behavior. We also discuss the potential use of players’ gameplay signatures which, in combination with recordings of how players truly behave in video cam sessions, can be used to establish evidence in the form of provable inconsistencies. The next step in this roadmap is for operators and the community to join efforts, establish open standards, and publish players’ reputation and risk, similar to the concept of a credit score, via an open and verifiable ledger.
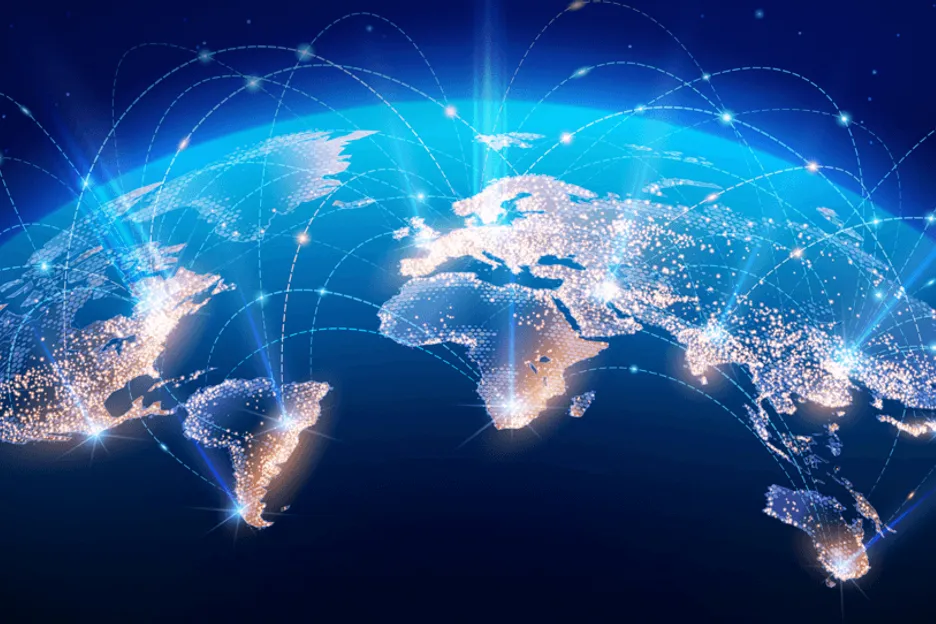
As opposed to the previous discussions, our thoughts on Future Solutions and the call for a more holistic revamp of the current centralized and inward-looking Web2.0 platform, smarter incentives, and the push for joint effort outside of the boundaries of individual operators is quite aspirational. Operators are likely to continue to run their business as usual, and they are maybe right to be reluctant because big changes will also have implications on the way they work with partners (for example, terms with affiliates and technology providers are often based on simple revenue-based metrics). Yet we believe these aspirations and long-term vision can help guide poker players. Our poker community is active, vibrant and influential. There are tons of smart and powerful opinion leaders who know the balance of power is in their favor and will leverage that to push the poker industry in the right direction.
How to Know If an Operator is Secure, Fair and Fun?
Poker has come a long way. Technology advances have made it possible for operators to successfully tackle security and fairness issues using powerful models for modeling value and risk. There is still a lot more to gain for both operators and players when the community works together towards adopting open standards, pushing for industry-wide identity and reputation, and working towards greater transparency. We conclude with what we think will push the operators in the right direction and help users gauge their level of investment and commitment:
- Ask them to publish independent tech reviews and certifications
- Ask them about the solutions and processes they have in place to identify bad actors
- Ask them to publish their list of bad actors
- Ask them to make cases public and supply material evidences
- Ask them to explain metrics and indicators used for establishing a player’s value profile, reputation
and risk - Ask them if you can export and use your value profile/reputation on other sites
If operators are not willing to come clean, are hand-wavy or lack plausibility and sophistication in answers to any of the above questions, we should take that as a clear signal for their lack of commitment and ability to ensure secure, fair, and fun games.
Read more about the mission to make online poker safe at A5labs.co. Over the next few weeks, Poker.Org will be running a series of articles around the issues and solutions detailed by A5 Labs, and we will be getting some of the biggest and most trusted poker players involved to debate the future of online poker.